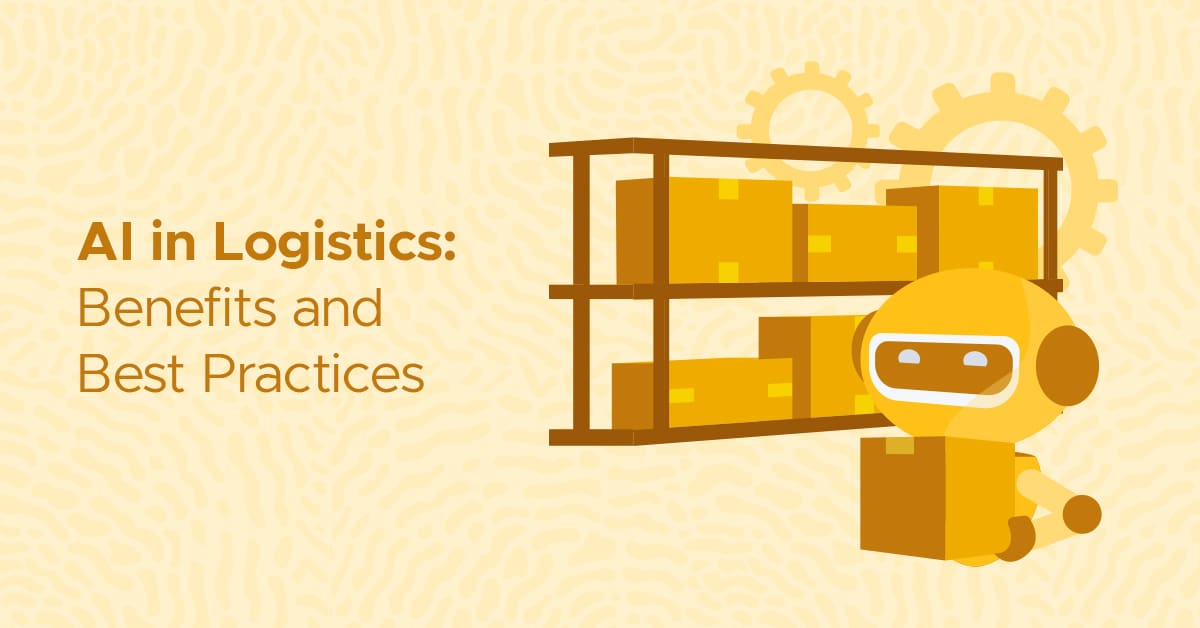
This is a guest post from Kate Parish. Kate is the CMO at Onilab, a web development agency. Being a top executive in a company specializing in eCommerce, she spends a lot of time researching new approaches to UX/UI design, Magento PWA development, and headless commerce. Kate also strives to keep up with the latest developments in digital marketing to modify the company’s promotional strategy accordingly. Her primary areas of interest are SEO, branding, and SMM.
Artificial intelligence (AI) is among the most discussed topics worldwide. The rapid development of generative AI has made this technology applicable to almost every aspect of our day-to-day activity, both work related and leisure. No wonder the abbreviation “AI” was Collins Dictionary’s Word of the Year for 2023.
However, AI in logistics is already familiar tool; it emerged way before ChatGPT caused a stir in society. With companies like Amazon using robots at local warehouses and others incorporating AI-powered sensors into vehicles, this technology has secured its position in supply chain management for years to come.
As the number of orders steadily grows and customers expect faster delivery speeds, you can’t hire a village to keep up with these demands, so implementing AI in logistics is a must.
In this article, we’ll look at the benefits of introducing AI in logistics. If you’re a start-up, you’ll learn how to gain a competitive advantage right from the get-go. Meanwhile, if you’re an established brand, you’ll discover how AI can help refine your operations.
AI is a broad term that encompasses several applications and techniques that serve various functions. In short, it’s aimed at mimicking human behavior.
Whether it’s machine learning that’s designed to improve with time or predictive analytics that forecasts future demand, AI is a versatile tool that can find its application in various spheres within the logistics industry.
Here is a closer look at how AI in logistics is revolutionizing supply chain management and logistics operations.
Machine learning (ML) algorithms parse large datasets to predict market trends. As it learns with each output, ML’s accuracy increases, which reduces logistical costs, enhances customer satisfaction, and optimizes every critical aspect of supply management.
These predictive capabilities make it an indispensable tool for boosting warehouse efficiency, refining the customer experience, and sifting through large amounts of data with minimal effort.
It can maintain optimal inventory levels, reduce the risk of overstocking or understocking, and forecast demand, among other advantages.
This AI in logistics solution relies on ML and data analytics to extract insights from warehouse equipment. For example, predictive analytics could alert a manager that their forklifts are about to break down.
Sensors and AI algorithms notice minor changes in equipment conditions. Then, they send a signal to the person in charge to help logistics companies foresee potential machine failures before they disrupt warehouse operations. They can even suggest scheduling maintenance proactively to avoid severe impacts on productivity.
Data continuously enters systems and frequently requires immediate action. For instance, stock levels can drop due to increased demand, so you’ll need to replenish inventory quickly.
AI systems analyze data upon arrival and support continuous operational flow in dynamic supply chains.
Say there’s something in the path of a delivery truck, such as traffic, and its AI-enabled sensor has observed it from a distance.
With AI in logistics, you can find the most efficient route to avoid delays regardless of external conditions like weather or road congestion.
Prescriptive analytics predicts outcomes, suggests courses of action, and shows the potential impact of each decision. It takes into account variables like cost, speed, and reliability to recommend the best shipping method or route.
Cognitive analytics focuses on unstructured data from various sources, including feedback, social media, and news feeds. It provides answers to complex questions in the form of dashboards and reports.
Descriptive analytics is a kind of data mining functionality that concentrates on examining massive datasets to find trends and produce summaries of what happened over a certain period, such as:
That information helps logistics companies derive insights to plan their future operations, resource allocation, and staffing needs.
Natural language processing (NLP) revolves around interpreting textual information and enabling the system to communicate with users like it’s a real person.
The AI-powered robot can answer questions, analyze the sentiment behind an input, and navigate the general context. As a result, you can hold a cohesive conversation, and the bot will refer to your previous responses to generate the best answer.
In logistics, NLP can be an asset in customer service by suggesting the best response to human agents or even replacing them entirely when dealing with simple inquiries.
It also plays a role in interpreting complex documentation such as international shipping regulations, invoices, and packing lists, thus reducing errors and increasing processing speed.
Image credit: Freepik
Proper inventory control ensures goods enter and exit a warehouse in the correct volume. That, in turn, assists with avoiding unexpected stockouts, insufficient inventory, and overstocking.
Order processing, picking, and packing are only a few variables involved in inventory management though. Further, these factors can make the process time-consuming and extremely prone to error.
This is where AI-driven supply chain planning systems and processes prove useful.
Thanks to their ability to manage large amounts of data, they can swiftly analyze and interpret information to provide timely supply chain and demand forecasting advice.
Some AI in logistics systems are so sophisticated, they can estimate seasonal demand and anticipate and identify new customer behavior patterns.
A well-functioning warehouse is essential in any supply chain; how quickly an order is assembled, packed, and dispatched shapes the customer experience and lays the groundwork for future purchases. AI implementation supports air-tight processes by:
If robots are trained on high-quality databases, logistical AI can minimize the instances of errors by 20% to 50% in your supply chain.
Suppose you employ AI for predictive analytics. If you spot an increased chance of malfunctioning ahead of time, you can take proactive measures to prevent potential harm to warehouse staff and equipment. If there is a danger, the system will make it known.
As far as security is concerned, AI can come in handy as both software and hardware protection.
For example, AI can scan your systems to detect suspicious login attempts. Or, consider the built-in AI-enabled scanners for surveillance cameras. Thanks to motion detection, facial recognition, and computer vision, such tools can minimize the risk of theft at your warehouses.
Automated systems can run without error for extended periods. Acting equally well in varied sectors, from customer service to the warehouse, AI lowers the number of workplace mishaps due to human oversight.
Further, warehouse robots can save on operating costs by attaining higher production levels through increased speed and precision.
Take utilizing them for picking and packing as an example: Under the guidance of AI, these autonomous machines learn the quickest and safest routes through the warehouse.
By evading various obstacles, they not only speed up operations but also reduce the risk of accidents (and thus human injury).
Other benefits of automated solutions include:
As a result, you save money on hiring more personnel and can instead invest it in educating your current workforce.
Plus, as employees free up more time, they can switch to more complex, value-added activities, cultivating a positive effect in the long run.
As mentioned earlier, AI in logistics saves valuable time and decreases dependency on manual efforts.
It contributes to faster, safer, and more intelligent processes so you deliver products to consumers’ destinations on time.
To meet shipping deadlines, automated systems speed up conventional warehouse processes and eliminate operational bottlenecks in the supply chain.
Image credit: Freepik
DHL analyzes data on 58 different parameters to build a machine-learning model for air freight. With this strategy, freight forwarders can determine up to a week in advance whether an increase or decrease in the average daily travel time is expected.
Another example is DHL’s platform: The company has developed their own AI-based product that can analyze public sentiment across social media and other online resources to spot possible issues with the supply chain.
The system leverages ML and NLP to comprehend the tone of online discussions and recognize possible shortages of materials, problems with access, and supplier status.
Amazon has successfully used autonomous vehicles for over a decade. After purchasing Kiva Systems (now Amazon Robotics) in 2012, the company now leverages robots at their warehouses to sort, pick, move, and stow orders in 26 of their 175 fulfillment centers.
UPS saves 10 million gallons of fuel a year with AI route optimization. That optimization cuts costs and enhances delivery efficiency, making UPS a leader in eco-friendly logistical practices.
IBM Watson has shown what AI vision can do through their device designed to recognize damaged train wagons with smart cameras.
Following their installation along train lines to capture the wagons, IBM Watson promptly collected and processed the information, ensuring operational reliability and the quality and safety of goods during transit.
The robot reached an accuracy rate of over 90% in its visual recognition tasks.
Mercedes-Benz (formerly known as Daimler) and other automotive corporations are pioneering platooning technology, which allows computers in autonomous vehicles to communicate with each other.
The goal is to ensure driving safety, decrease fuel consumption, and cover longer distances without stopping to refill gas tanks.
So, you’ve decided to follow the example of logistics companies using AI. Let’s break down some essential steps in this process.
Logistics and supply chain organizations should start small and focus on one specific task to complete with AI.
For example, you could begin with route optimization or introducing a chatbot in customer support. This approach provides a controlled setting to experiment with AI in logistics to identify patterns and obtain accurate predictions.
Once you determine through your pilot project where and how AI can significantly lower operational costs and improve data quality, you can proceed to roll it out and scale the system across other parts of the supply chain.
The bottom line: By gradually integrating AI, you avoid disrupting established operations.
One of the main (and most difficult) tasks of integrating AI in logistics is marrying it to your current systems and frameworks.
Your workers are used to long-established rules and practices, so they may be reluctant to change what already works.
Add to this the headaches associated with incorporating an AI tool into dozens of tracking, communication, and management platforms.
To make this process more manageable and avoid incompatibility, be sure to:
Give logistics AI full access to big data. “Big” entails several criteria, including not only the huge volume of data pouring into your systems but also the speed of its accumulation and a diversity of formats.
In other words, data is big when it’s impossible for humans to put it to good use, but AI can do it with ease.
Imagine extending these achievements by integrating other efficient features into your logistical systems.
Suppose you utilize Salesforce to track interactions; by adding tools like Salesforce CPQ and AI, you can generate accurate pricing variations and create a seamless flow from customer inquiry to finalized service delivery.
That’s where you need to understand the Salesforce CPQ benefits clearly and the ways to enhance it with AI.
Everyone should understand the purpose of having to change their approach to supply chain management and utilizing AI in logistics.
Moreover, when people don’t know how to use new tools properly and they end up hampering their productivity, they’ll soon ditch the innovation.
To prevent that pushback, significant investment in workforce training is required. Employees should know how to apply AI-generated insights to their work, whether it’s historical data analysis or predictive analysis.
Encourage continuous learning and adaptation to help you fully leverage AI’s capabilities, as well as be prepared for changes and ongoing AI developments.
AI promises to revolutionize global supply chains and logistics. That being said, it would be unfair to say that the AI future in supply chain optimization is trouble-free. So, let’s construct a holistic view of leveraging AI in logistics organizations.
Most AI systems are hosted on the cloud and need a lot of bandwidth, so logistical service providers may need to purchase specialized hardware to function optimally.
If you’re a budding supply chain partner, that cost can be a significant up-front investment, not to mention hefty setup expenditures.
Cloud-based and AI technologies are built to be scaled up. As more information is accumulated, the system will need to adjust to the growing load.
Considering this, AI service providers price their products high enough to handle more complex operations, which may be too expensive for smaller logistics companies.
However, this obstacle is gradually fading with the proliferation of AI-as-a-Service, which makes the technology more accessible.
Such an approach lets logistics providers introduce AI in phases, targeting specific operational needs for immediate benefits and a clearer path to ROI.
The proper implementation of AI requires staff training, which entails a sizable time and financial commitment. Supply chain companies will have to collaborate closely with AI providers to develop an effective and reasonably priced training program.
The initial cost involves purchasing proprietary hardware or sophisticated software licenses. Additionally, every component of an AI-operated device requires maintenance and the replacement of consumables.
Owing to potential energy and cost implications, the operational investment may be relatively substantial.
When it’s time to replace any of those components, utility rates may skyrocket, which could immediately affect overhead costs.
AI is transforming the shipping practices we’re used to. It excels in various tasks, offering route optimization, higher quality data, real-time tracking, and lower operational costs.
In the upcoming years, AI start-ups and logistics companies will need to cooperate to produce more state-of-the-art innovation. Now’s the time to tap into this sphere and make AI an indispensable ally on your road to logistical success.